VivadoAccelerator Backend
The VivadoAccelerator
backend of hls4ml
leverages the PYNQ software stack to easily deploy models on supported devices.
Currently hls4ml
supports the following boards:
pynq-z2 (part:
xc7z020clg400-1
)zcu102 (part:
xczu9eg-ffvb1156-2-e
)alveo-u50 (part:
xcu50-fsvh2104-2-e
)alveo-u250 (part:
xcu250-figd2104-2L-e
)alveo-u200 (part:
xcu200-fsgd2104-2-e
)alveo-u280 (part:
xcu280-fsvh2892-2L-e
)
but, in principle, support can be extended to any board supported by PYNQ. For the Zynq-based boards, there are two components: an ARM-based processing system (PS) and FPGA-based programmable logic (PL), with various intefaces between the two.
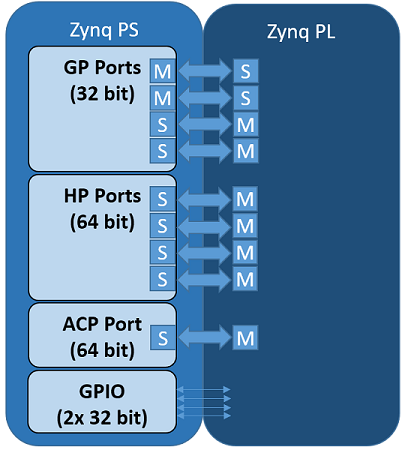
Neural Network Overlay
In the PYNQ project, programmable logic circuits are presented as hardware libraries called overlays.
The overlay can be accessed through a Python API.
In hls4ml
, we create a custom neural network overlay, which sends and receives data via AXI stream.
The target device is programmed using a bitfile that is generated by the VivadoAccelerator
backend.
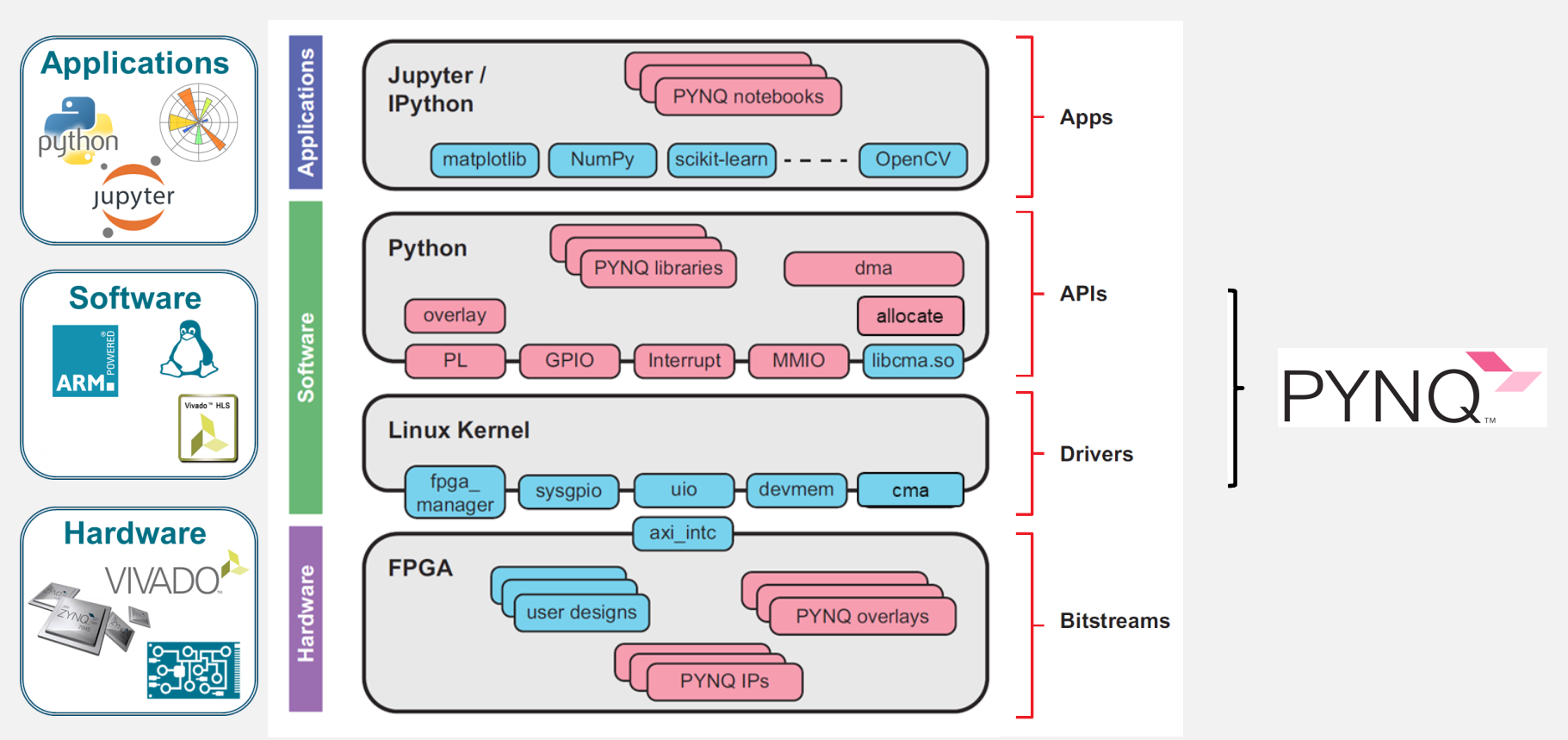
Example
This example is taken from part 7 of the hls4ml tutorial.
Specifically, we’ll deploy a model on a pynq-z2
board.
First, we generate the bitfile from a Keras model model
and a config.
import hls4ml
config = hls4ml.utils.config_from_keras_model(model, granularity='name')
hls_model = hls4ml.converters.convert_from_keras_model(model,
hls_config=config,
output_dir='hls4ml_prj_pynq',
backend='VivadoAccelerator',
board='pynq-z2')
hls4ml.build(bitfile=True)
After this command completes, we will need to package up the bitfile, hardware handoff, and Python driver to copy to the PS of the board.
mkdir -p package
cp hls4ml_prj_pynq/myproject_vivado_accelerator/project_1.runs/impl_1/design_1_wrapper.bit package/hls4ml_nn.bit
cp hls4ml_prj_pynq/myproject_vivado_accelerator/project_1.srcs/sources_1/bd/design_1/hw_handoff/design_1.hwh package/hls4ml_nn.hwh
cp hls4ml_prj_pynq/axi_stream_driver.py package/
tar -czvf package.tar.gz -C package/ .
Then we can copy this package to the PS of the board and untar it.
Finally, on the PS in Python we can create a NeuralNetworkOverlay
object, which will download the bitfile onto the PL of the board.
We also must provide the shapes of our input and output data, X_test.shape
and y_test.shape
, respectively, to allocate the buffers for the data transfer.
The predict
method will send the input data to the PL and return the output data y_hw
.
from axi_stream_driver import NeuralNetworkOverlay
nn = NeuralNetworkOverlay('hls4ml_nn.bit', X_test.shape, y_test.shape)
y_hw, latency, throughput = nn.predict(X_test, profile=True)